Онлайн книга
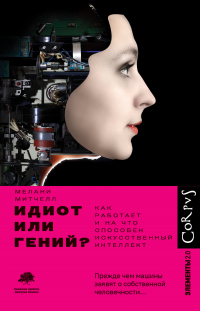
Примечания книги
1
A. Cuthbertson, “DeepMind AlphaGo: AI Teaches Itself «Thousands of Years of Human Knowledge» Without Help”, Newsweek, Oct. 18, 2017, www.newsweek.com/deepmind-alphago-ai-teaches-human-help-687620.
2
Здесь и далее я цитирую высказывания Дугласа Хофштадтера из интервью, которое я взяла у него после встречи в Google, причем цитаты точно отражают содержание и тон его ремарок, сделанных в присутствии инженеров Google.
3
Слова Джека Шварца цит. по: G.-C. Rota, Indiscrete Thoughts (Boston: Berkhäuser, 1997), 22.
4
D. R. Hofstadter, Gödel, Escher, Bach: an Eternal Golden Braid (New York: Basic Books, 1979), 678. (Русское издание: Хофштадтер Д. Гёдель. Эшер. Бах: эта бесконечная гирлянда / Пер. с англ. М. Эскиной. – Самара: Бахрах-М, 2001.)
5
Ibid., 676.
6
Цит. по: D. R. Hofstadter, “Staring Emmy Straight in the Eye – and Doing My Best Not to Flinch”, in Creativity, Cognition, and Knowledge, ed. T. Dartnell (Westport, Conn.: Praeger, 2002), 67–100.
7
Цит. по: R. Cellan-Jones, “Stephen Hawking Warns Artificial Intelligence Could End Mankind”, BBC News, Dec. 2, 2014, www.bbc.com/news/technology-30290540.
8
M. McFarland, “Elon Musk: «With Artificial Intelligence, We Are Summoning the Demon»”, Washington Post, Oct. 24, 2014. https://www.washingtonpost.com/news/innovations/wp/2014/10/24/elon-musk-with-artificial-intelligence-we-are-summoning-the-demon/
9
Bill Gates, on Reddit, Jan. 28, 2015, www.reddit.com/r/IAmA/comments/2tzjp7/hi_reddit_im_bill_gates_and_im_back_for_my_third/?.
10
Бостром Н. Искусственный интеллект: Этапы. Угрозы. Стратегии / Пер. с англ. С. Филина. – М.: Манн, Иванов и Фербер, 2016. (Здесь и далее в сносках, если не указано иное, – прим. перев.)
11
Цит. по: K. Anderson, “Enthusiasts and Skeptics Debate Artificial Intelligence”, Vanity Fair, Nov. 26, 2014.
12
R. A. Brooks, “Mistaking Performance for Competence”, in What to Think About Machines That Think, ed. J. Brockman (New York: Harper Perennial, 2015), 108–111.
13
Цит. по: G. Press, “12 Observations About Artificial Intelligence from the O’Reilly AI Conference”, Forbes, Oct. 31, 2016, www.forbes.comobservations-about-artificial-intelligence-from-the-oreilly-ai-conference/sites/gilpress/2016/10/31/12-/#886a6012ea2e.
14
J. McCarthy et al., “A Proposal for the Dartmouth Summer Research Project in Artificial Intelligence”, submitted to the Rockefeller Foundation, 1955, reprinted in AI Magazine 27, no. 4 (2006): 12–14.
15
Кибернетика – это междисциплинарная наука, которая изучает закономерности “управления и коммуникации в живых организмах и машинах”. См. N. Wiener, Cybernetics (Cambridge, Mass.: MIT Press, 1961).
16
Цит. по: N. J. Nilsson, John McCarthy: A Biographical Memoir (Washington, D. C.: National Academy of Sciences, 2012).
17
McCarthy et al., “Proposal for the Dartmouth Summer Research Project in Artificial Intelligence”.
18
Ibid.
19
G. Solomonoff, Ray Solomonoff and the Dartmouth Summer Research Project in Artificial Intelligence, 1956, accessed Dec. 4, 2018, www.raysolomonoff.com/dartmouth/dartray.pdf.
20
H. Moravic, Mind Children: The Future of Robot and Human Intelligence (Cambridge, Mass.: Harvard University Press, 1988), 20.
21
H. A. Simon, The Shape of Automation for Men and Management (New York: Harper & Row, 1965), 96.
22
M. L. Minsky, Computation: Finite and Infinite Machines (Upper Saddle River, N. J.: Prentice-Hall, 1967), 2.
23
B. R. Redman, The Portable Voltaire (New York: Penguin Books, 1977), 225.
24
M. L. Minsky, The Emotion Machine: Commonsense Thinking, Artificial Intelligence, and the Future of the Human Mind (New York: Simon & Schuster, 2006), 95.
25
One Hundred Year Study on Artificial Intelligence (AI100), 2016 Report, 13, ai100.stanford.edu/2016-report.
26
Ibid., 12.
27
J. Lehman, J. Clune and S. Risi, “An Anarchy of Methods: Current Trends in How Intelligence Is Abstracted in AI”, IEEE Intelligent Systems 29, no. 6 (2014): 56–62.
28
A. Newell and H. A. Simon, “GPS: A Program That Simulates Human Thought”, P-2257, Rand Corporation, Santa Monica, Calif. (1961).
29
F. Rosenblatt, “The Perceptron: A Probabilistic Model for Information Storage and Organization in the Brain”, Psychological Review 65, no. 6 (1958): 386–408.
30
Математически алгоритм обучения перцептрона описывается следующим образом. Для каждого веса wj: wj ← wj + η (t – y) xj, где t – верный выходной сигнал (1 или 0) для заданного входного сигнала, y – фактический выходной сигнал перцептрона, xj – входной сигнал, связанный с весом wj, а η – скорость обучения, задаваемая программистом. Стрелка обозначает обновление. Порог учитывается путем создания дополнительного “входного сигнала” x0 с постоянным значением 1, которому присваивается вес w0 = –порог. При наличии этого дополнительного входного сигнала и веса (называемого смещением) перцептрон дает сигнал на выходе, только если сумма входных сигналов, помноженных на веса (то есть скалярное произведение входного вектора и вектора веса) больше или равняется 0. Часто входные значения масштабируются и подвергаются другим преобразованиям, чтобы веса не становились слишком велики.
31
Цит. по: M. Olazaran, “A Sociological Study of the Official History of the Perceptrons Controversy”, Social Studies of Science 26, no. 3 (1996): 611–659.
32
M. A. Boden, Mind as Machine: A History of Cognitive Science (Oxford: Oxford University Press, 2006), 2:913.
33
M. L. Minsky and S. L. Papert, Perceptrons: An Introduction to Computational Geometry (Cambridge, Mass.: MIT Press, 1969). (Минский М., Пейперт С. Персептроны / Пер. с англ. Г. Гимельфарба и В. Шарыпанова – М.: Издательство “Мир”, 1971.)
34
Выражаясь техническим языком, любую булеву функцию можно вычислить с помощью полностью подключенной многослойной сети с линейными пороговыми значениями и одним внутренним (“скрытым”) слоем.
35
Olazaran, “Sociological Study of the Official History of the Perceptrons Controversy”.
36
G. Nagy, “Neural Networks – Then and Now”, IEEE Transactions on Neural Networks 2, no. 2 (1991): 316–318.
37
Minsky and Papert, “Perceptrons”, 231–232. (Пер. с англ. Г. Гимельфарба и В. Шарыпанова.)
38
J. Lighthill, “Artificial Intelligence: A General Survey”, in Artificial Intelligence: A Paper Symposium (London: Science Research Council, 1973).
39
Цит. по: C. Moewes and A. Nürnberger, Computational Intelligence in Intelligent Data Analysis (New York: Springer, 2013), 135.
40
M. L. Minsky, The Society of Mind (New York: Simon & Schuster, 1987), 29.
41
Активация y для каждой скрытой и выходной единицы обычно определяется как логистическая функция от скалярного произведения векторов x (входные сигналы единицы) и w (веса связей этой единицы): y = 1/ (1 + e– (x⋅w)). Векторы x и w также включают “смещение” и активацию. Если единицы имеют нелинейные выходные функции, например сигмоиды, при наличии достаточного количества скрытых единиц сеть может вычислить любую функцию (с минимальными ограничениями) при любом желаемом уровне аппроксимации. Этот факт называется универсальной теоремой аппроксимации. Подробнее см. в работе M. Nielsen, Neural Networks and Deep Learning, neuralnetworksanddeeplearning.com.
42
Для читателей, знакомых с математическим анализом: метод обратного распространения ошибки – это разновидность градиентного спуска, при которой для каждого веса w в сети вычисляется приблизительное направление самого крутого спуска на “поверхности ошибки”. Это направление вычисляется путем определения градиента функции ошибок (например, квадрата разницы выходного сигнала и контрольного значения) по отношению к весу w. Рассмотрим, например, вес w связи входной единицы i со скрытой единицей h. Вес w корректируется в направлении самого крутого спуска на величину, определяемую ошибкой, которая была распространена на единицу h, а также активацией единицы i и задаваемой пользователем скоростью обучения. Если вы хотите лучше изучить метод обратного распространения ошибки, рекомендую обратиться к бесплатной онлайн-книге Michael Nielsen, Neural Networks and Deep Learning.
43
В моей сети с 324 входными сигналами, 50 скрытыми единицами и 10 выходными сигналами 324 × 50 = 16 200 весов у связей входных сигналов со скрытым слоем и 50 × 10 = 500 весов у связей скрытого слоя с выходным слоем, что в сумме дает 16 700 весов.
44
D. E. Rumelhart, J. L. McClelland and the PDP Research Group, Parallel Distributed Processing: Explorations in the Microstructure of Cognition (Cambridge, Mass.: MIT Press, 1986), 1:3.
45
Ibid., 113.
46
Цит. по: C. Johnson, “Neural Network Startups Proliferate Across the U. S”., The Scientist, Oct. 17, 1988.
47
A. Clark, Being There: Putting Brain, Body, and World Together Again (Cambridge, Mass.: MIT Press, 1996), 26.
48
Как подсказал мне Дуглас Хофштадтер, грамматически правильнее было бы называть его Good Old Old-Fashioned AI (“старым добрым старомодным ИИ”), но аббревиатуре GOOFAI не хватает лаконичности GOFAI.
49
Q. V. Le et al., “Building High-Level Features Using Large-Scale Unsupervised Learning”, in Proceedings of the International Conference on Machine Learning (2012), 507–514.
50
P. Hoffman, “Retooling Machine and Man for Next Big Chess Faceoff”, New York Times, Jan. 21, 2003.
51
D. L. McClain, “Chess Player Says Opponent Behaved Suspiciously”, New York Times, Sept. 28, 2006.
52
Цит. по: M. Y. Vardi, “Artificial Intelligence: Past and Future”, Communications of the Association for Computing Machinery 55, no. 1 (2012): 5.
53
K. Kelly, “The Three Breakthroughs That Have Finally Unleashed AI on the World”, Wired, Oct. 27, 2014.
54
J. Despres, “Scenario: Shane Legg”, Future, accessed Dec. 4, 2018, future.wikia.com/wiki/Scenario: _Shane_Legg.
55
Цит. по: H. McCracken, “Inside Mark Zuckerberg’s Bold Plan for the Future of Facebook”, Fast Company, Nov. 16, 2015, www.fastcompany.com/3052885/mark-zuckerberg-facebook.
56
V. C. Müller and N. Bostrom, “Future Progress in Artificial Intelligence: A Survey of Expert Opinion”, in Fundamental Issues of Artificial Intelligence, ed. V. C. Müller (Cham, Switzerland: Springer International, 2016), 555–572.
57
M. Loukides and B. Lorica, “What Is Artificial Intelligence?”, O’Reilly, June 20, 2016, www.oreilly.com/ideas/what-is-artificial-intelligence.
58
S. Pinker, “Thinking Does Not Imply Subjugating”, in What to Think About Machines That Think, ed. J. Brockman (New York: Harper Perennial, 2015), 5–8.
59
A. M. Turing, “Computing Machinery and Intelligence”, Mind 59, no. 236 (1950): 433–460.
60
J. R. Searle, “Minds, Brains, and Programs”, Behavioral and Brain Sciences 3, no. 3 (1980): 417–424.
61
J. R. Searle, Mind: A Brief Introduction (Oxford: Oxford University Press, 2004), 66.
62
Термины “сильный ИИ” и “слабый ИИ” также используются в значении “общий ИИ” и “ограниченный ИИ”. Именно так их использует Рэй Курцвейл, но Сёрл изначально давал им другие определения.
63
Статья Сёрла воспроизводится в книге D. R. Hofstadter and D. C. Dennett, The Mind’s I: Fantasies and Reflections on Self and Soul (New York: Basic Books, 1981) и сопровождается убедительным контраргументом Хофштадтера.
64
S. Aaronson, Quantum Computing Since Democritus (Cambridge, U. K.: Cambridge University Press, 2013), 33.
65
“Turing Test Transcripts Reveal How Chatbot «Eugene» Duped the Judges”, Coventry University, June 30, 2015, www.coventry.ac.uk/primary-news/turing-test-transcripts-reveal-how-chatbot-eugene-duped-the-judges/.
66
“Turing Test Success Marks Milestone in Computing History”, University of Reading, June 8, 2014, www.reading.ac.uk/news-and-events/releases/PR583836.aspx.
67
R. Kurzweil, The Singularity Is Near: When Humans Transcend Biology (New York: Viking Press, 2005), 7.
68
Ibid., 22–23.
69
I. J. Good, “Speculations Concerning the First Ultraintelligent Machine”, Advances in Computers 6 (1966): 31–88.
70
V. Vinge, “First Word”, Omni, Jan. 1983.
71
Kurzweil, Singularity Is Near, 241, 317, 198–199.
72
B. Wang, “Ray Kurzweil Responds to the Issue of Accuracy of His Predictions”, Next Big Future, Jan. 19, 2010, www.nextbigfuture.com/2010/01/ray-kurzweil-responds-to-issue-of.html.
73
D. Hochman, “Reinvent Yourself: The Playboy Interview with Ray Kurzweil”, Playboy, April 19, 2016, www.playboy.com/articles/playboy-interview-ray-kurzweil.
74
Kurzweil, Singularity Is Near, 136.
75
A. Kreye, “A John Henry Moment”, in Brockman, What to Think About Machines That Think, 394–396.
76
Kurzweil, Singularity Is Near, 494.
77
R. Kurzweil, “A Wager on the Turing Test: Why I Think I Will Win”, Kurzweil AI, April 9, 2002, www.kurzweilai.net/a-wager-on-the-turing-test-why-i-think-i-will-win.
78
Ibid.
79
Ibid.
80
Ibid.
81
M. Dowd, “Elon Musk’s Billion-Dollar Crusade to Stop the A. I. Apocalypse”, Vanity Fair, March 26, 2017.
82
L. Grossman, “2045: The Year Man Becomes Immortal”, Time, Feb. 10, 2011.
83
From Singularity University website, accessed Dec. 4, 2018, su.org/about/.
84
Kurzweil, Singularity Is Near, 316.
85
R. Kurzweil, The Age of Spiritual Machines: When Computers Exceed Human Intelligence (New York: Viking Press, 1999), 170.
86
D. R. Hofstadter, “Moore’s Law, Artificial Evolution, and the Fate of Humanity”, in Perspectives on Adaptation in Natural and Artificial Systems, ed. L. Booker et al. (New York: Oxford University Press, 2005), 181.
87
Все цитаты взяты из книги Kurzweil, Age of Spiritual Machines, 169–170.
88
Hofstadter, “Moore’s Law, Artificial Evolution, and the Fate of Humanity”, 182.
89
From Long Bets website: longbets.org/about.
90
From Long Bets website, Bet 1: longbets.org/1/#adjudication_terms.
91
Ibid.
92
Ibid.
93
Kurzweil, “Wager on the Turing Test”.
94
M. Kapor, “Why I Think I Will Win”, Kurzweil AI, April 9, 2002, http://www.kurzweilai.net/why-i-think-i-will-win.
95
Ibid.
96
R. Kurzweil, foreword to Virtual Humans, by P. M. Plantec (New York: AMACOM, 2004).
97
Цит. по: Grossman, “2045”.
98
S. A. Papert, “The Summer Vision Project”, MIT Artificial Intelligence Group Vision Memo 100 (July 7, 1966), dspace.mit.edu/handle/1721.1/6125.
99
D. Crevier, AI: The Tumultuous History of the Search for Artificial Intelligence (New York: Basic Books, 1993), 88.
100
K. Fukushima, “Cognitron: A Self-Organizing Multilayered Neural Network Model”, Biological Cybernetics 20, no. 3–4 (1975): 121–36; K. Fukushima, “Neocognitron: A Hierarchical Neural Network Capable of Visual Pattern Recognition”, Neural Networks 1, no. 2 (1988): 119–130.
101
Прежде чем загружать изображение в сеть, его необходимо привести к фиксированному размеру, соответствующему размеру первого слоя сети.
102
При описании работы мозга, как правило, приходится делать много оговорок, и мои слова не исключение. Хотя я описала процесс относительно точно, мозг невероятно сложен, а перечисленные открытия позволяют лишь частично понять механизм зрения, многое в котором до сих пор остается загадкой для ученых.
103
Массив весов для каждой карты признаков называется сверточным фильтром, или ядром свертки.
104
Здесь я использую термин “модуль классификации” в качестве условного обозначения того, что обычно называется полносвязными слоями глубокой сверточной нейронной сети.
105
Я многое опускаю в своем описании сверточных нейронных сетей. Например, чтобы вычислить свою активацию, ячейка слоя свертки выполняет свертку, а затем применяет к результату нелинейную функцию активации. Кроме того, в сверточных нейронных сетях, как правило, бывают также слои другого типа, называемые пулинговыми слоями. Подробнее см. в работе I. Goodfellow, Y. Bengio and A. Courville, Deep Learning (Cambridge, Mass.: MIT Press, 2016).
106
В момент написания этой книги открыть “поиск по картинкам” Google можно на images.google.com, нажав на маленькую иконку в форме фотоаппарата в поисковой строке.
107
Алгоритм обратного распространения ошибки одновременно разработали несколько групп, и долгое время исследователи нейронных сетей спорили, кому принадлежит заслуга его создания.
108
Цит. по: D. Hernandez, “Facebook’s Quest to Build an Artificial Brain Depends on This Guy”, Wired, Aug. 14, 2014, www.wired.com/2014/08/deep-learning-yann-lecun/.
109
PASCAL (Pattern Analysis, Statisctical Modelling and Computational Learning) – Анализ шаблонов, статистическое моделирование и машинное обучение.
110
Кроме того, проходило состязание в “обнаружении”, в рамках которого программам необходимо было также локализовать объекты разных категорий на изображениях, и другие специальные состязания, но здесь я рассматриваю именно состязание в классификации.
111
D. Gershgorn, “The Data That Transformed AI Research – and Possibly the World”, Quartz, July 26, 2017, qz.com/1034972/the-data-that-changed-the-direction-of-ai-research-and-possibly-the-world/.
112
“About Amazon Mechanical Turk”, www.mturk.com/help.
113
L. Fei-Fei and J. Deng, “ImageNet: Where Have We Been? Where Are We Going?”, slides at image-net.org/challenges/talks_2017/imagenet_ilsvrc2017_v1.0.pdf.
114
A. Krizhevsky, I. Sutskever and G. E. Hinton, “ImageNet Classification with Deep Convolutional Neural Networks”, Advances in Neural Information Processing Systems 25 (2012): 1097–1105.
115
T. Simonite, “Teaching Machines to Understand Us”, Technology Review, Aug. 5, 2015, www.technologyreview.com/s/540001/teaching-machines-to-understand-us/.
116
ImageNet Large Scale Visual Recognition Challenge announcement, June 2, 2015, www.image-net.org/challenges/LSVRC/announcement-June-2–2015.
117
S. Chen, “Baidu Fires Scientist Responsible for Breaching Rules in High-Profile Supercomputer AI Test”, South China Morning Post, international edition, June 12, 2015, www.scmp.com/tech/science-research/article/1820649/chinas-baidu-fires-researcher-after-team-cheated-high-profile.
118
Gershgorn, “Data That Transformed AI Research”.
119
Цит. по: Hernandez, “Facebook’s Quest to Build an Artificial Brain Depends on This Guy”.
120
B. Agüera y Arcas, “Inside the Machine Mind: Latest Insights on Neuroscience and Computer Science from Google” (lecture video), Oxford Martin School, May 10, 2016, www.youtube.com/watch?v=v1dW7ViahEc.
121
K. He et al., “Delving Deep into Rectifiers: Surpassing Human-Level Performance on ImageNet Classification”, in Proceedings of the IEEE International Conference on Computer Vision (2015), 1026–1034.
122
A. Linn, “Microsoft Researchers Win ImageNet Computer Vision Challenge”, AI Blog, Microsoft, Dec. 10, 2015, blogs.microsoft.com/ai/2015/12/10/microsoft-researchers-win-imagenet-computer-vision-challenge.
123
A. Hern, “Computers Now Better than Humans at Recognising and Sorting Images”, Guardian, May 13, 2015, www.theguardian.com/global/2015/may/13/baidu-minwa-supercomputer-better-than-humans-recognising-images; T. Benson, “Microsoft Has Developed a Computer System That Can Identify Objects Better than Humans”, UPI, Feb. 14, 2015, www.upi.com/Science_News/2015/02/14/Microsoft-has-developed-a-computer-system-that-can-identify-objects-better-than-humans/1171423959603.
124
A. Karpathy, “What I Learned from Competing Against a ConvNet on ImageNet”, Sept. 2, 2014, karpathy.github.io/2014/09/02/what-i-learned-from-competing-against-a-convnet-on-imagenet.
125
S. Lohr, “A Lesson of Tesla Crashes? Computer Vision Can’t Do It All Yet”, New York Times, Sept. 19, 2016.
126
Читатели, следившие за ходом американских президентских выборов 2016 года, заметят перекличку с лозунгом сторонников Берни Сандерса Feel the Bern (“Почувствуй огонь Берни”).
127
E. Brynjolfsson and A. McAfee, “The Business of Artificial Intelligence”, Harvard Business Review, July 2017.
128
O. Tanz, “Can Artificial Intelligence Identify Pictures Better than Hu-mans?”, Entrepreneur, April 1, 2017, www.entrepreneur.com/article/283990.
129
D. Vena, “3 Top AI Stocks to Buy Now”, Motley Fool, March 27, 2017, www.fool.comtop-ai-stocks-to-buy-now.aspx/investing/2017/03/27/3-.
130
Цит. по: C. Metz, “A New Way for Machines to See, Taking Shape in Toronto”, New York Times, Nov. 28, 2017, www.nytimes.com/2017/11/28/technology/artificial-intelligence-research-toronto.html.
131
Цит. по: J. Tanz, “Soon We Won’t Program Computers. We’ll Train Them Like Dogs”, Wired, May 17, 2016.
132
Из выступления Гарри Шума на Исследовательском саммите Microsoft в Редмонде (штат Вашингтон), июнь 2017 года.
133
Подробнее об этом в работе J. Lanier, Who Owns the Future? (New York: Simon & Schuster, 2013).
134
Tesla’s Customer Privacy Policy, accessed Dec. 7, 2018, www.tesla.com/about/legal.
135
T. Bradshaw, “Self-Driving Cars Prove to Be Labour-Intensive for Humans”, Financial Times, July 8, 2017.
136
“Ground Truth Datasets for Autonomous Vehicles”, Mighty AI, accessed Dec. 7, 2018, mty.ai/adas/.
137
“Deep Learning in Practice: Speech Recognition and Beyond”, EmTech Digital video, May 23, 2016, events.technologyreview.com/emtech/digital/16/video/watch/andrew-ng-deep-learning.
138
Y. Bengio, “Machines That Dream”, in The Future of Machine Intelligence: Perspectives from Leading Practitioners, ed. D. Beyer (Sebastopol, Calif.: O’Reilly Media), 14.
139
W. Landecker et al., “Interpreting Individual Classifications of Hierarchical Networks”, in Proceedings of the 2013 IEEE Symposium on Computational Intelligence and Data Mining (2013), 32–38.
140
M. R. Loghmani et al., “Recognizing Objects in-the-Wild: Where Do We Stand?”, in IEEE International Conference on Robotics and Automation (2018), 2170–2177.
141
H. Hosseini et al., “On the Limitation of Convolutional Neural Networks in Recognizing Negative Images”, in Proceedings of the 16th IEEE International Conference on Machine Learning and Applications (2017), 352–358; R. Geirhos et al., “Generalisation in Humans and Deep Neural Networks”, Advances in Neural Information Processing Systems 31 (2018): 7549–7561; M. Alcorn et al., “Strike (with) a Pose: Neural Networks Are Easily Fooled by Strange Poses of Familiar Objects”, arXiv:1811.11553 (2018).
142
M. Orcutt, “Are Face Recognition Systems Accurate? Depends on Your Race”, Technology Review, July 6, 2016, www.technologyreview.com/s/601786/are-face-recognition-systems-accurate-depends-on-your-race.
143
J. Zhao et al., “Men Also Like Shopping: Reducing Gender Bias Amplification Using Corpus-Level Constraints”, in Proceedings of the 2017 Conference on Empirical Methods in Natural Language Processing (2017).
144
W. Knight, “The Dark Secret at the Heart of AI”, Technology Review, April 11, 2017, www.technologyreview.com/s/604087/the-dark-secret-at-the-heart-of-ai/.
145
C. Szegedy et al., “Intriguing Properties of Neural Networks”, in Proceedings of the International Conference on Learning Representations (2014).
146
A. Nguyen, J. Yosinski and J. Clune, “Deep Neural Networks Are Easily Fooled: High Confidence Predictions for Unrecognizable Images”, in Proceedings of the IEEE Conference on Computer Vision and Pattern Recognition (2015), 427–436.
147
См., например, M. Mitchell, An Introduction to Genetic Algorithms (Cambridge, Mass.: MIT Press, 1996).
148
Nguyen, Yosinski and Clune, “Deep Neural Networks Are Easily Fooled”.
149
M. Sharif et al., “Accessorize to a Crime: Real and Stealthy Attacks on State-of-the-Art Face Recognition”, in Proceedings of the 2016 ACM SIGSAC Conference on Computer and Communications Security (2016), 1528–1540.
150
K. Eykholt et al., “Robust Physical-World Attacks on Deep Learning Visual Classification”, in Proceedings of the IEEE Conference on Computer Vision and Pattern Recognition (2018), 1625–1634.
151
S. G. Finlayson et al., “Adversarial Attacks on Medical Machine Learning”, Science 363, no. 6433 (2019): 1287–1289.
152
Цит. по: W. Knight, “How Long Before AI Systems Are Hacked in Creative New Ways?”, Technology Review, Dec. 15, 2016, www.technologyreview.com/s/603116/how-long-before-ai-systems-are-hacked-in-creative-new-ways.
153
J. Clune, “How Much Do Deep Neural Networks Understand About the Images They Recognize?”, lecture slides (2016), accessed Dec. 7, 2018, c4dm.eecs.qmul.ac.uk/horse2016/HORSE2016_Clune.pdf.
154
Цит. по: D. Palmer, “AI Could Help Solve Humanity’s Biggest Issues by Taking Over from Scientists, Says DeepMind CEO”, Computing, May 26, 2015, www.computing.co.uk/ctg/news/2410022/ai-could-help-solve-humanity-s-biggest-issues-by-taking-over-from-scientists-says-deepmind-ceo.
155
S. Lynch, “Andrew Ng: Why AI Is the New Electricity”, Insights by Stanford Business, March 11, 2017, www.gsb.stanford.edu/insights/andrew-ng-why-ai-new-electricity.
156
J. Anderson, L. Rainie and A. Luchsinger, “Artificial Intelligence and the Future of Humans”, Pew Research Center, Dec. 10, 2018, www.pewinternet.org/2018/12/10/artificial-intelligence-and-the-future-of-humans.
157
Вот две недавние книги об этических проблемах, связанных с ИИ и большими данными: C. O’Neil, Weapons of Math Destruction: How Big Data Increases Inequality and Threatens Democracy (New York: Crown, 2016); H. Fry, Hello World: Being Human in the Age of Algorithms (New York: W. W. Norton, 2018). (Русское издание: Фрай Х. Hello World: Как быть человеком в эпоху машин / Пер. с англ. Ю. Плискиной. М.: Corpus, 2020.)
158
C. Domonoske, “Facebook Expands Use of Facial Recognition to ID Users in Photos”, National Public Radio, Dec. 19, 2017, www.npr.org/sections/thetwo-way/2017/12/19/571954455/facebook-expands-use-of-facial-recognition-to-id-users-in-photos.
159
H. Hodson, “Face Recognition Row over Right to Identify You in the Street”, New Scientist, June 19, 2015.
160
J. Snow, “Amazon’s Face Recognition Falsely Matched 28 Members of Congress with Mugshots”, Free Future (blog), ACLU, July 26, 2018, www.aclu.org/blog/privacy-technology/surveillance-technologies/amazons-face-recognition-falsely-matched-28.
161
B. Brackeen, “Facial Recognition Software Is Not Ready for Use by Law Enforcement”, Tech Crunch, June 25, 2018, techcrunch.com/2018/06/25/facial-recognition-software-is-not-ready-for-use-by-law-enforcement.
162
B. Smith, “Facial Recognition Technology: The Need for Public Regulation and Corporate Responsibility”, Microsoft on the Issues (blog), Microsoft, July 13, 2018, blogs.microsoft.com/on-the-issues/2018/07/13/facial-recognition-technology-the-need-for-public-regulation-and-corporate-responsibility.
163
K. Walker, “AI for Social Good in Asia Pacific”, Around the Globe (blog), Google, Dec. 13, 2018, www.blog.google/around-the-globe/google-asia/ai-social-good-asia-pacific.
164
B. Goodman and S. Flaxman, “European Union Regulations on Algorithmic Decision-Making and a «Right to Explanation»”, AI Magazine 38, no. 3 (Fall 2017): 50–57.
165
“Article 12, EU GDPR: Transparent Information, Communication, and Modalities for the Exercise of the Rights of the Data Subject”, EU General Data Protection Regulation, accessed Dec. 7, 2018, www.privacy-regulation.eutransparent-information-communication-and-modalities-for-the-exercise-of-the-rights-of-the-data-subject-GDPR.htm/en/article-12-.
166
Partnership on AI website, accessed Dec. 18, 2018, www.partnershiponai.org.
167
Подробнее об этом см. в работе W. Wallach and C. Allen, Moral Machines: Teaching Robots Right from Wrong (New York: Oxford University Press, 2008).
168
I. Asimov, I, Robot (Bantam Dell, 2004), 37. (First edition: Grove, 1950.)
169
A. C. Clarke, 2001: A Space Odyssey (London: Hutchinson & Co, 1968).
170
Ibid., 192. Цитируется в переводе Я. Берлина и Н. Галь.
171
N. Wiener, “Some Moral and Technical Consequences of Automation”, Science 131, no. 3410 (1960): 1355–1358.
172
J. J. Thomson, “The Trolley Problem”, Yale Law Journal 94, no. 6 (1985): 1395–1415.
173
См., например, J. Achenbach, “Driverless Cars Are Colliding with the Creepy Trolley Problem”, Washington Post, December 29, 2015.
174
J.-F. Bonnefon, A. Shariff, and I. Rahwan, “The Social Dilemma of Autonomous Vehicles”, Science 352, no. 6293 (2016): 1573–1576.
175
J. D. Greene, “Our Driverless Dilemma”, Science 352, no. 6293 (2016): 1514–15.
176
См., например, M. Anderson and S. L. Anderson, “Machine Ethics: Creating an Ethical Intelligent Agent”, AI Magazine 28, no. 4 (2007): 15.
177
A. Sutherland, “What Shamu Taught Me About a Happy Marriage”, New York Times, June 25, 2006, www.nytimes.com/2006/06/25/fashion/what-shamu-taught-me-about-a-happy-marriage.html.
178
thejetsons.wikia.com/wiki/Rosey.
179
Этот метод обучения с подкреплением, называемый обучением по значениям (value learning), не единственный. Цель второго метода – обучения на основе правил поведения (policy learning) – состоит в том, чтобы непосредственно узнавать, какое действие необходимо выполнять в конкретном состоянии, вместо того чтобы сначала выяснять числовые оценки действий.
180
C. J. Watkins and P. Dayan, “Q-Learning”, Machine Learning 8, nos. 3–4 (1992): 279–292.
181
Подробное техническое введение в обучение с подкреплением см. в работе R. S. Sutton and A. G. Barto, Reinforcement Learning: An Introduction, 2nd ed. (Cambridge, Mass.: MIT Press, 2017), incompleteideas.net/book/the-book-2nd.html.
182
См., например, следующие статьи: P. Christiano et al., “Transfer from Simulation to Real World Through Learning Deep Inverse Dynamics Model”, arXiv:1610.03518 (2016); J. P. Hanna and P. Stone, “Grounded Action Transformation for Robot Learning in Simulation”, in Proceedings of the Conference of the American Association for Artificial Intelligence (2017), 3834–3840; A. A. Rusu et al., “Sim-to-Real Robot Learning from Pixels with Progressive Nets”, in Proceedings of the First Annual Conference on Robot Learning, CoRL (2017); S. James, A. J. Davison and E. Johns, “Transferring End-to-End Visuomotor Control from Simulation to Real World for a Multi-stage Task”, in Proceedings of the First Annual Conference on Robot Learning, CoRL (2017); M. Cutler, T. J. Walsh and J. P. How, “Real-World Reinforcement Learning via Multifidelity Simulators”, IEEE Transactions on Robotics 31, no. 3 (2015): 655–671.
183
Слова Демиса Хассабиса цит. по: P. Iwaniuk, “A Conversation with Demis Hassabis, the Bullfrog AI Prodigy Now Finding Solutions to the World’s Big Problems”, PCGamesN, accessed Dec. 7, 2018, www.pcgamesn.com/demis-hassabis-interview.
184
Цит. по: “From Not Working to Neural Networking”, Economist, June 25, 2016.
185
M. G. Bellemare et al., “The Arcade Learning Environment: An Evaluation Platform for General Agents”, Journal of Artificial Intelligence Research 47 (2013): 253–279.
186
Точнее, программа DeepMind использовала так называемый эпсилон-жадный алгоритм, чтобы выбирать действие на каждом шаге. При вероятности ε программа выбирает действие случайным образом, а при вероятности (1 – ε) – действие с самой высокой оценкой. Эпсилон – это величина в диапазоне от 0 до 1; сначала она близка к 1 и постепенно уменьшается в ходе эпизодов обучения.
187
R. S. Sutton and A. G. Barto, Reinforcement Learning: An Introduction, 2nd ed. (Cambridge, Mass.: MIT Press, 2017), 124, incompleteideas.net/book/the-book-2nd.html.
188
Подробнее см. в работе V. Mnih et al., “Human-Level Control Through Deep Reinforcement Learning”, Nature 518, no. 7540 (2015): 529.
189
V. Mnih et al., “Playing Atari with Deep Reinforcement Learning”, Proceedings of the Neural Information Processing Systems (NIPS) Conference, Deep Learning Workshop (2013).
190
“Arthur Samuel”, History of Computers website, history-computer.com/ModernComputer/thinkers/Samuel.html.
191
“Машинное обучение” в XX в. не было частью “искусственного интеллекта”, а противопоставлялось ему как направление, основанное на анализе примеров, а не на логическом выводе, в отличие от программы Сэмюэла. Вопреки расхожему мнению, публикация Сэмюэла не содержала никаких утверждений о том, что “машинное обучение – это такая область исследований, которая дает компьютерам способность обучаться вещам, не запрограммированным заранее”. Российские специалисты по ИИ считают, что общепринятое понимание машинного обучения было введено и популяризовано московским ученым Э. М. Браверманом (1931–1977) в его книге “Обучение машины распознаванию образов”, опубликованной им совместно с журналистом А. Г. Аркадьевым в 1964 г. в издательстве “Наука”. Этот тезис был неоднократно высказан, не встретив возражений, на международной конференции “Бравермановские чтения”, прошедшей 28–30 апреля 2017 г. в Бостоне. (См. L. Rozonoer, B. Mirkin, I. Muchnik (Eds.). Braverman Readings in Machine Learning. State-of-the-Art Survey, LNAI 11100, Springer, 2018). (Прим. науч. ред.)
192
Программа Сэмюэла использовала переменное число слоев в зависимости от хода.
193
Программа Сэмюэла также на каждом своем ходе применяла метод альфа-бета-отсечения, чтобы определить, какие узлы дерева игры не требуют оценки. Альфа-бета-отсечение также активно использовалось шахматной программой Deep Blue, разработанной в IBM.
194
Подробнее см. в работе A. L. Samuel, “Some Studies in Machine Learning Using the Game of Checkers”, IBM Journal of Research and Development 3, no. 3 (1959): 210–229.
195
Ibid.
196
J. Schaeffer et al., “CHINOOK: The World Man-Machine Checkers Champion”, AI Magazine 17, no. 1 (1996): 21.
197
D. Hassabis, “Artificial Intelligence: Chess Match of the Century”, Nature 544 (2017): 413–414.
198
A. Newell, J. Calman Shaw and H. A. Simon, “Chess-Playing Programs and the Problem of Complexity”, IBM Journal of Research and Development 2, no. 4 (1958): 320–335.
199
M. Newborn, Deep Blue: An Artificial Intelligence Milestone (New York: Springer, 2003), 236.
200
Цит. по: J. Goldsmith, “The Last Human Chess Master”, Wired, Feb. 1, 1995.
201
Цит. по: M. Y. Vardi, “Artificial Intelligence: Past and Future”, Communications of the Association for Computing Machinery 55, no. 1 (2012): 5.
202
A. Levinovitz, “The Mystery of Go, the Ancient Game That Computers Still Can’t Win”, Wired, May 12, 2014.
203
G. Johnson, “To Test a Powerful Computer, Play an Ancient Game”, New York Times, July 29, 1997.
204
Цит. по: “S. Korean Go Player Confident of Beating Google’s AI”, Yonhap News Agency, Feb. 23, 2016, english.yonhapnews.co.kr/search1/2603000000.html?cid=AEN20160223003651315.
205
Цит. по: M. Zastrow, “«I’m in Shock!»: How an AI Beat the World’s Best Human at Go”, New Scientist, March 9, 2016, www.newscientist.comim-in-shock-how-an-ai-beat-the-worlds-best-human-at-go/article/2079871-.
206
C. Metz, “The Sadness and Beauty of Watching Google’s AI Play Go”, Wired, March 11, 2016, www.wired.com/2016/03/sadness-beauty-watching-googles-ai-play-go.
207
“For Artificial Intelligence to Thrive, It Must Explain Itself”, Economist, Feb. 15, 2018, www.economist.comif-it-cannot-who-will-trust-it-artificial-intelligence-thrive-it-must/news/science-and-technology/21737018-.
208
P. Taylor, “The Concept of «Cat Face»”, London Review of Books, Aug. 11, 2016.
209
Цит. по: S. Byford, “DeepMind Founder Demis Hassabis on How AI Will Shape the Future”, Verge, March 10, 2016, www.theverge.com/2016/3/10/11192774/demis-hassabis-interview-alphago-google-deepmind-ai.
210
D. Silver et al., “Mastering the Game of Go Without Human Knowledge”, Nature, 550 (2017): 354–59.
211
D. Silver et al., “A General Reinforcement Learning Algorithm That Masters Chess, Shogi, and Go Through Self-Play”, Science 362, no. 6419 (2018): 1140–1144.
212
Цит. по: P. Iwaniuk, “A Conversation with Demis Hassabis, the Bullfrog AI Prodigy Now Finding Solutions to the World’s Big Problems”, PCGamesN, accessed Dec. 7, 2018, www.pcgamesn.com/demis-hassabis-interview.
213
E. David, “DeepMind’s AlphaGo Mastered Chess in Its Spare Time”, Silicon Angle, Dec. 6, 2017, siliconangle.com/blog/2017/12/06/deepminds-alphago-mastered-chess-spare-time.
214
Так, если не выходить за пределы игровой сферы, в 2018 году DeepMind опубликовала статью, описывающую систему обучения с подкреплением, которая, по утверждению компании, демонстрировала некоторую способность к переносу обучения игре в разные видеоигры Atari. L. Espeholt et al., “Impala: Scalable Distributed Deep-RL with Importance Weighted Actor-Learner Architectures”, in Proceedings of the International Conference on Machine Learning (2018), 1407–1416.
215
D. Silver et al., “Mastering the Game of Go Without Human Knowledge”, Nature 550 (2017): 354–359.
216
G. Marcus, “Innateness, AlphaZero, and Artificial Intelligence”, arXiv:1801.05667 (2018).
217
F. P. Such et al., “Deep Neuroevolution: Genetic Algorithms Are a Competitive Alternative for Training Deep Neural Networks for Reinforcement Learning”, Proceedings of the Neural Information Processing Systems (NIPS) Conference, Deep Reinforcement Learning Workshop (2018).
218
M. Mitchell, An Introduction to Genetic Algorithms (Cambridge, Mass.: MIT Press, 1996).
219
Marcus, “Innateness, AlphaZero, and Artificial Intelligence”.
220
G. Marcus, “Deep Learning: A Critical Appraisal”, arXiv:1801.00631 (2018).
221
K. Kansky et al., “Schema Networks: Zero-Shot Transfer with a Generative Causal Model of Intuitive Physics”, in Proceedings of the International Conference on Machine Learning (2017), 1809–1818.
222
A. A. Rusu et al., “Progressive Neural Networks”, arXiv:1606.04671 (2016).
223
Marcus, “Deep Learning”.
224
Цит. по: N. Sonnad and D. Gershgorn, “Q&A: Douglas Hofstadter on Why AI Is Far from Intelligent”, Quartz, Oct. 10, 2017, qz.com/1088714/qa-douglas-hofstadter-on-why-ai-is-far-from-intelligent.
225
Стоит отметить, что несколько робототехнических групп разработали роботов для загрузки посудомоечных машин, но, насколько мне известно, для обучения этих роботов не использовалось ни обучение с подкреплением, ни какой-либо другой метод машинного обучения. На впечатляющие способности этих роботов можно посмотреть на видео (например, “Robotic Dog Does Dishes, Plays Fetch”, NBC New York, June 23, 2016, www.nbcnewyork.com/news/local/Boston-Dynamics-Dog-Does-Dishes-Brings-Sodas-384140021.html), но очевидно, что они пока довольно ограниченны и не могут решить споров насчет мытья посуды, которые каждый вечер разгораются в моей семье.
226
A. Karpathy, “AlphaGo, in Context”, Medium, May 31, 2017, medium.com./@karpathy/alphago-in-context-c47718cb95a5
227
Я написала историю о ресторане, ориентируясь на подобные короткие истории, созданные Роджером Шэнком и его коллегами при работе над пониманием естественного языка (R. C. Schank and C. K. Riesbeck, Inside Computer Understanding: Five Programs Plus Miniatures [Hillsdale, N. J.: Lawrence Erlbaum Associates, 1981]) и Джоном Сёрлом в его критике ИИ (J. R. Searle, “Minds, Brains, and Programs”, Behavioral and Brain Sciences 3, no. 3 [1980]: 417–424).
228
G. Hinton et al., “Deep Neural Networks for Acoustic Modeling in Speech Recognition: The Shared Views of Four Research Groups”, IEEE Signal Processing Magazine 29, no. 6 (2012): 82–97.
229
J. Dean, “Large Scale Deep Learning”, slides from keynote lecture, Conference on Information and Knowledge Management (CIKM), Nov. 2014, accessed Dec. 7, 2018, static.googleusercontent.com/media/research.google.com/en//people/jeff/CIKM-keynote-Nov2014.pdf.
230
S. Levy, “The iBrain Is Here, and It’s Already in Your Phone”, Wired, Aug. 24, 2016, www.wired.com/2016/08/an-exclusive-look-at-how-ai-and-machine-learning-work-at-apple.
231
В литературе по распознаванию речи в качестве метрики производительности чаще всего используется “вероятность ошибки в кодовом слове” (word error rate, WER) в больших наборах коротких аудиосегментов. Хотя при оценке на основе этих наборов WER современных систем распознавания речи находится на “человеческом уровне” или превосходит его, существует ряд причин утверждать, что при проведении оценки на основе более реалистичных фрагментов (например, содержащих шумы, акценты, значимые слова, неоднозначный язык) производительность машинного распознавания речи по-прежнему значительно уступает человеческой. Хороший обзор некоторых аргументов на этот счет приводится в работе A. Hannun, “Speech Recognition Is Not Solved”, accessed Dec. 7, 2018, awni.github.io/speech-recognition.
232
Хорошее, хотя и техническое, описание работы современных алгоритмов распознавания речи дается в работе J. H. L. Hansen and T. Hasan, “Speaker Recognition by Machines and Humans: A Tutorial Review”, IEEE Signal Processing Magazine 32, no. 6 (2015): 74–99.
233
Отзывы взяты с сайта Amazon.com и незначительно отредактированы в некоторых случаях.
234
На момент написания этих строк онлайн-мир еще не оправился от новости, что компания Cambridge Analytica, занимавшаяся анализом данных, использовала данные десятков миллионов пользователей Facebook для таргетирования политической рекламы, вероятно, применяя среди прочих техник и анализ тональности текстов.
235
Кодер – отличается от более универсального термина “кодировщик”. (Прим. науч. ред.)
236
Как вы помните из главы 2, каждая единица нейронной сети вычисляет математическую функцию суммы своих входных сигналов, умноженных на их веса. Это возможно только при численных входных сигналах.
237
J. Firth, “A Synopsis of Linguistic Theory, 1930–1955”, in Studies in Linguistic Analysis (Oxford: Philological Society, 1957), 1–32.
238
A. Lenci, “Distributional Semantics in Linguistic and Cognitive Research”, Italian Journal of Linguistics 20, no. 1 (2008): 1–31.
239
В физике понятие “вектор” часто определяется как сущность, имеющая длину и направление. Это определение эквивалентно тому, которое я дала в тексте: любой вектор может быть уникальным образом описан координатами точки, где длина – это длина отрезка от начала координат до этой точки, а направление – угол наклона этого отрезка относительно осей координат.
240
T. Mikolov et al., “Efficient Estimation of Word Representations in Vector Space”, in Proceedings of the International Conference on Learning Representations (2013).
241
Word2vec, Google Code Archive, code.google.com/archive/p/word2vec/. Контекстные векторы также называются векторными представлениями слов.
242
Здесь я иллюстрирую вариацию метода skip-gram – одного из двух предложенных в работе Mikolov et al., “Efficient Estimation of Word Representations in Vector Space”.
243
Ibid.
244
Для получения этих результатов я использовала демоверсию word2vec на bionlp-www.utu.fi/wv_demo/ (с применением модели English Google-News Negative300).
245
Суть в том, чтобы найти x в векторном уравнении man – woman = king – x. Для сложения и вычитания векторов просто складывайте и вычитайте соответствующие элементы, например: (3, 2, 4) – (1, 1, 1) = = (2, 1, 3).
246
http://bionlp-www.utu.fi/wv_demo/.
247
R. Kiros et al., “Skip-Thought Vectors”, in Advances in Neural Information Processing Systems 28 (2015), 3294–3302.
248
Цит. по: H. Devlin, “Google a Step Closer to Developing Machines with Human-Like Intelligence”, Guardian, May 21, 2015, www.theguardian.com/science/2015/may/21/google-a-step-closer-to-developing-machines-with-human-like-intelligence.
249
Y. LeCun, “What’s Wrong with Deep Learning?”, lecture slides, p. 77, accessed Dec. 14, 2018, www.pamitc.orgcvpr-keynote.pdf/cvpr15/files/lecun-20150610-.
250
См., например, T. Bolukbasi et al., “Man Is to Computer Programmer as Woman Is to Homemaker? Debiasing Word Embeddings”, in Advances in Neural Information Processing Systems 29 (2016), 4349–4357.
251
См., например, J. Zhao et al., “Learning Gender-Neutral Word Embeddings”, in Proceedings of the 2018 Conference on Empirical Methods in Natural Language Processing (2018), 4847–4853, и A. Sutton, T. Lansdall-Welfare and N. Cristianini, “Biased Embeddings from Wild Data: Measuring, Understanding, and Removing”, in Proceedings of the International Symposium on Intelligent Data Analysis (2018), 328–339.
252
Q. V. Le and M. Schuster, “A Neural Network for Machine Translation, at Production Scale”, AI Blog, Google, Sept. 27, 2016, ai.google-blog.com/2016/09/a-neural-network-for-machine.html.
253
W. Weaver, “Translation”, in Machine Translation of Languages, ed. W. N. Locke and A. D. Booth (New York: Technology Press and John Wiley & Sons, 1955), 15–23.
254
“Google Переводчик” применяет этот метод для большинства языков. На момент написания книги “Google Переводчик” еще не перешел к использованию нейронных сетей для некоторых менее распространенных языков.
255
Подробнее см. в работе Y. Wu et al., “Google’s Neural Machine Translation System: Bridging the Gap Between Human and Machine Translation”, arXiv:1609.08144 (2016).
256
В системе нейронного машинного перевода Google контекстные векторы определяются в ходе обучения всей сети.
257
Точнее, выходные сигналы сети-декодера – это вероятности для каждого возможного слова из лексикона сети (здесь – французского). Подробнее см. в работе Wu et al., “Google’s Neural Machine Translation System”.
258
На момент написания этой книги “Google Переводчик” и другие переводческие системы переводят по одному предложению. Пример исследования перевода, который отходит от этой схемы, приводится в работе L. M. Werlen and A. Popescu-Belis, “Using Coreference Links to Improve Spanish-to-English Machine Translation”, in Proceedings of the 2nd Workshop on Coreference Resolution Beyond OntoNotes (2017), 30–40.
259
S. Hochreiter and J. Schmidhuber, “Long Short-Term Memory”, Neural Computation 9, no. 8 (1997): 1735–1780.
260
Wu et al., “Google’s Neural Machine Translation System”.
261
Ibid.
262
T. Simonite, “Google’s New Service Translates Languages Almost as Well as Humans Can”, Technology Review, Sept. 27, 2016, www.technologyreview.com/s/602480/googles-new-service-translates-languages-almost-as-well-as-humans-can.
263
A. Linn, “Microsoft Reaches a Historic Milestone, Using AI to Match Human Performance in Translating News from Chinese to English”, AI Blog, Microsoft, March 14, 2018, blogs.microsoft.com/ai/machine-translation-news-test-set-human-parity.
264
“IBM Watson Is Now Fluent in Nine Languages (and Counting)”, Wired, Oct. 6, 2016, www.wired.co.uk/article/connecting-the-cognitive-world.
265
A. Packer, “Understanding the Language of Facebook”, EmTech Digital video lecture, May 23, 2016, events.technologyreview.com/video/watch/alan-packer-understanding-language.
266
DeepL Pro, press release, March 20, 2018, www.deepl.com/press.html.
267
K. Papineni et al., “BLEU: A Method for Automatic Evaluation of Machine Translation”, in Proceedings of the 40th Annual Meeting of the Association for Computational Linguistics (2002), 311–318.
268
Wu et al., “Google’s Neural Machine Translation System”; H. Hassan et al., “Achieving Human Parity on Automatic Chinese to English News Translation”, arXiv:1803.05567 (2018).
269
Выполненный “Google Переводчиком” перевод истории о ресторане на французский язык: Un homme est entré dans un restaurant et a commandé un hamburger, cuit rare. Quand il est arrivé, il a été brûlé à un croustillant. La serveuse s’arrêta devant la table de l’homme. “Est-ce que le hamburger va bien?” demanda-t-elle. “Oh, c’est génial”, dit l’homme en repoussant sa chaise et en sortant du restaurant sans payer. La serveuse a crié après lui, “Hé, et le projet de loi?” Elle haussa les épaules, marmonnant dans son souffle, “Pourquoi est-il si déformé?”
270
Выполненный “Google Переводчиком” перевод истории о ресторане на итальянский язык: Un uomo andò in un ristorante e ordinò un hamburger, cucinato raro. Quando è arrivato, è stato bruciato per un croccante. La cameriera si fermò accanto al tavolo dell’uomo. “L’hamburger va bene?” Chiese lei. “Oh, è semplicemente fantastico”, disse l’uomo, spingendo indietro la sedia e uscendo dal ristorante senza pagare. La cameriera gli urlò dietro, “Ehi, e il conto?” Lei scrollò le spalle, mormorando sottovoce, “Perché è così piegato?”
271
Выполненный “Google Переводчиком” перевод истории о ресторане на китайский язык: —名男子走进—家 餐厅, 点了—个罕见的汉堡包. 当它到达时, 它被烧得脆脆. 女服务员停在男人的桌 子旁边. “汉堡好吗” 她问. “哦, 这太好了”, 那男人说, 推开椅子, 没有付钱就冲出餐 厅. 女服务员大声喊道:“嘿, 账单呢?” 她耸了耸, 低声嘀咕道, “他为什么这么弯腰?”
272
Подробнее о проблемах, связанных с отсутствием понимания у “Google Переводчика”, см. в работе D. R. Hofstadter, “The Shallowness of Google Translate”, The Atlantic, Jan. 30, 2018.
273
D. R. Hofstadter, Gödel, Escher, Bach: an Eternal Golden Braid (New York: Basic Books, 1979), 603.
274
E. Davis and G. Marcus, “Commonsense Reasoning and Commonsense Knowledge in Artificial Intelligence”, Communications of the ACM 58, no. 9 (2015): 92–103.
275
O. Vinyals et al., “Show and Tell: A Neural Image Caption Generator”, in Proceedings of the IEEE Conference on Computer Vision and Pattern Recognition (2015), 3156–3164; A. Karpathy and L. Fei-Fei, “Deep Visual-Semantic Alignments for Generating Image Descriptions”, in Proceedings of the IEEE Conference on Computer Vision and Pattern Recognition (2015), 3128–3137.
276
На рис. 39 приводится схема упрощенной версии системы, описанной в работе Vinyals et al., “Show and Tell”.
277
J. Markoff, “Researchers Announce Advance in Image-Recognition Software”, New York Times, Nov. 17, 2014.
278
J. Walker, “Google’s AI Can Now Caption Images Almost as Well as Humans”, Digital Journal, Sept. 23, 2016, www.digitaljournal.comaccuracy/tech-and-science/technology/google-s-ai-now-captions-images-with-94-/article/475547.
279
A. Linn, “Picture This: Microsoft Research Project Can Interpret, Caption Photos”, AI Blog, May 28, 2015, blogs.microsoft.com/ai/picture-this-microsoft-research-project-can-interpret-caption-photos.
280
Microsoft CaptionBot, www.captionbot.ai.
281
Transcript from www.chakoteya.net/NextGen/130.htm.
282
Цит. по: F. Manjoo, “Where No Search Engine Has Gone Before”, Slate, April 11, 2013, www.slate.com/articles/technology/technology/2013/04/google_has_a_single_towering_obsession_it_wants_to_build_the_star_trek_computer.html.
283
Цит. по: C. Thompson, “What Is I. B. M.’s Watson?”, New York Times Magazine, June 16, 2010.
284
Цит. по: K. Johnson, “How «Star Trek» Inspired Amazon’s Alexa”, Venture Beat, June 7, 2017, venturebeat.com/2017/06/07/how-star-trek-inspired-amazons-alexa.
285
Jeopardy! – прообраз российской “Своей игры”.
286
Wikipedia, s.v. “Watson (computer)”, accessed Dec. 16, 2018, en.wikipedia.org/wiki/Watson_ (computer).
287
Thompson, “What Is I. B. M.’s Watson?”
288
Этот мем пришел из мультсериала “Симпсоны”.
289
K. Jennings, “The Go Champion, the Grandmaster, and Me”, Slate, March 15, 2016, www.slate.com/articles/technology/technology/2016/03/google_s_alphago_defeated_go_champion_lee_sedol_ken_jennings_explains_what.html.
290
Английская идиома to push the envelope (букв. “толкнуть конверт”) имеет значение “выйти за рамки, сломать стереотипы”.
291
Ответ составлен из названия шоколадного батончика Baby Ruth и имени судьи Верховного суда Рут Гинзбург.
292
Цит. по: D. Kawamoto, “Watson Wasn’t Perfect: IBM Explains the «Jeopardy!» Errors”, Aol, accessed Dec. 16, 2018, www.aol.com/2011/02/17/the-watson-supercomputer-isnt-always-perfect-you-say-tomato.
293
J. C. Dvorak, “Was IBM’s Watson a Publicity Stunt from the Start?”, PC Magazine, Oct. 30, 2013, www.pcmag.com/article2/0,2817,2426521,00.asp.
294
M. J. Yuan, “Watson and Healthcare”, IBM Developer website, April 12, 2011, www.ibm.com/developerworks/library/os-ind-watson/index.html.
295
“Artificial Intelligence Positioned to Be a Game-Changer”, 60 Minutes, Oct. 9, 2016, www.cbsnews.comminutes-artificial-intelligence-charlie-rose-robot-sophia/news/60-.
296
C. Ross and I. Swetlitz, “IBM Pitched Its Watson Supercomputer as a Revolution in Cancer Care. It’s Nowhere Close”, Stat News, Sept. 5, 2017, www.statnews.com/2017/09/05/watson-ibm-cancer.
297
P. Rajpurkar et al., “SQuAD: 100,000+ Questions for Machine Comprehension of Text”, in Proceedings of the 2016 Conference on Empirical Methods in Natural Language Processing (2016), 2383–2392.
298
Ibid.
299
A. Linn, “Microsoft Creates AI That Can Read a Document and Answer Questions About It as Well as a Person”, AI Blog, Microsoft, Jan. 15, 2018, blogs.microsoft.com/ai/microsoft-creates-ai-can-read-document-answer-questions-well-person.
300
Цит. по: “AI Beats Humans at Reading Comprehension for the First Time”, Technology.org, Jan. 17, 2018, www.technology.org/2018/01/17/ai-beats-humans-at-reading-comprehension-for-the-first-time.
301
D. Harwell, “AI Models Beat Humans at Reading Comprehension, but They’ve Still Got a Ways to Go”, Washington Post, Jan. 16, 2018.
302
P. Clark et al., “Think You Have Solved Question Answering? Try ARC, the AI2 Reasoning Challenge”, arXiv:1803.05457 (2018).
303
Ibid.
304
ARC Dataset Leaderboard, Allen Institute for Artificial Intelligence, accessed Dec. 17, 2018, leaderboard.allenai.org/arc/submissions/public.
305
Все приведенные в настоящем разделе примеры взяты из работы E. Davis, L. Morgenstern and C. Ortiz, “The Winograd Schema Challenge”, accessed Dec. 17, 2018, cs.nyu.edu/faculty/davise/papers/WS.html.
306
T. Winograd, Understanding Natural Language (New York: Academic Press, 1972).
307
H. J. Levesque, E. Davis and L. Morgenstern, “The Winograd Schema Challenge”, in AAAI Spring Symposium: Logical Formalizations of Commonsense Reasoning (American Association for Artificial Intelligence, 2011), 47.
308
T. H. Trinh and Q. V. Le, “A Simple Method for Commonsense Reasoning”, arXiv:1806.02847 (2018).
309
Цит. по: K. Bailey, “Conversational AI and the Road Ahead”, Tech Crunch, Feb. 25, 2017, techcrunch.com/2017/02/25/conversational-ai-and-the-road-ahead.
310
H. Chen et al., “Attacking Visual Language Grounding with Adversarial Examples: A Case Study on Neural Image Captioning”, in Proceedings of the 56th Annual Meeting of the Association for Computational Linguistics, vol. 1, Long Papers (2018), 2587–2597.
311
N. Carlini and D. Wagner, “Audio Adversarial Examples: Targeted Attacks on Speech-to-Text”, in Proceedings of the First Deep Learning and Security Workshop (2018).
312
R. Jia and P. Liang, “Adversarial Examples for Evaluating Reading Comprehension Systems”, in Proceedings of the 2017 Conference on Empirical Methods in Natural Language Processing (2017).
313
C. D. Manning, “Last Words: Computational Linguistics and Deep Learning”, Nautilus, April 2017.
314
G.-C. Rota, “In Memoriam of Stan Ulam: The Barrier of Meaning”, Physica D Nonlinear Phenomena 22 (1986): 1–3.
315
Однажды я читала лекцию на эту тему, и студент задал мне вопрос: “Зачем системе ИИ человеческое понимание? Почему мы не можем принять ИИ с другим типом понимания?” Во-первых, мне сложно представить “другой тип понимания”, а во-вторых, я считаю, что системы ИИ должны понимать ситуации, с которыми сталкиваются, примерно так же, как их понимают люди, поскольку предполагается, что эти системы будут взаимодействовать с людьми в реальном мире.
316
Понятие “изначальное знание” активно используют психолог Элизабет Спелке и ее коллеги; см. E. S. Spelke and K. D. Kinzler, “Core Knowledge”, Developmental Science 10, no. 1 (2007): 89–96. Подобные идеи высказывают и многие другие специалисты по когнитивной психологии.
317
Психологи пользуются понятием “интуитивный”, поскольку это базовое знание с раннего возраста укореняется в нашем разуме, становится для нас самоочевидным и остается по большей части бессознательным. Многие психологи при этом продемонстрировали ошибочность некоторых аспектов интуитивных представлений человека о физике, вероятности и других вещах. См., например, A. Tversky and D. Kahneman, “Judgment Under Uncertainty: Heuristics and Biases”, Science 185, no. 4157 (1974): 1124–31; and B. Shanon, “Aristotelianism, Newtonianism, and the Physics of the Layman”, Perception 5, no. 2 (1976): 241–243.
318
Лоуренс Барсалоу подробно описывает такие ментальные симуляции в работе L. W. Barsalou, “Perceptual Symbol Systems”, Behavioral and Brain Sciences 22 (1999): 577–660.
319
Дуглас Хофштадтер отмечает, что, когда человек сталкивается с ситуацией (либо вспоминает, читает или размышляет о ней), репрезентация ситуации в его разуме включает также “ореол” возможных вариаций этой ситуации, который он называет “имплицитной контрафактуальной сферой”. В него входят “вещи, которые никогда не происходили, но которые мы все равно не можем не видеть”. D. R. Hofstadter, Metamagical Themas (New York: Basic Books, 1985), 247.
320
L. W. Barsalou, “Grounded Cognition”, Annual Review of Psychology 59 (2008): 617–645.
321
L. W. Barsalou, “Situated Simulation in the Human Conceptual System”, Language and Cognitive Processes 18, no. 5–6 (2003): 513–562.
322
A. E. M. Underwood, “Metaphors”, Grammarly (blog), accessed Dec. 17, 2018, www.grammarly.com/blog/metaphor.
323
Шекспир У. “Ромео и Джульетта”. Перевод Б. Пастернака.
324
Шекспир У. “Макбет”. Перевод М. Лозинского.
325
G. Lakoff and M. Johnson, Metaphors We Live By (Chicago: University of Chicago Press, 1980).
326
L. E. Williams and J. A. Bargh, “Experiencing Physical Warmth Promotes Interpersonal Warmth”, Science 322, no. 5901 (2008): 606–607.
327
C. B. Zhong and G. J. Leonardelli, “Cold and Lonely: Does Social Exclusion Literally Feel Cold?”, Psychological Science 19, no. 9 (2008): 838–842.
328
D. R. Hofstadter, I Am a Strange Loop (New York: Basic Books, 2007). Цитата с переднего клапана суперобложки. Мое описание также перекликается с идеями, которые философ Дэниел Деннет предложил в своей книге Consciousness Explained (New York: Little, Brown, 1991).
329
Такая “лингвистическая продуктивность” обсуждается в работах D. Hofstadter and E. Sander, Surfaces and Essences: Analogy as the Fuel and Fire of Thinking (New York: Basic Books, 2013), 129, и A. M. Zwicky and G. K. Pullum, “Plain Morphology and Expressive Morphology”, in Annual Meeting of the Berkeley Linguistics Society (1987), 13: 330–340.
330
Я позаимствовала этот аргумент из настоящего судебного дела. См. “Blogs as Graffiti? Using Analogy and Metaphor in Case Law”, IdeaBlawg, March 17, 2012, www.ideablawg.ca/blog/2012/3/17/blogs-as-graffiti-using-analogy-and-metaphor-in-case-law.html.
331
D. R. Hofstadter, “Analogy as the Core of Cognition”, Presidential Lecture, Stanford University (2009), accessed Dec. 18, 2018, www.youtube.com/watch?v=n8m7lFQ3njk.
332
Hofstadter and Sander, Surfaces and Essences, 3.
333
M. Minsky, “Decentralized Minds”, Behavioral and Brain Sciences 3, no. 3 (1980): 439–440.
334
D. B. Lenat and J. S. Brown, “Why AM and EURISKO Appear to Work”, Artificial Intelligence 23, no. 3 (1984): 269–294.
335
Примеры взяты из статьи C. Metz, “One Genius’ Lonely Crusade to Teach a Computer Common Sense”, Wired, March 24, 2016, www.wired.com/2016/03/doug-lenat-artificial-intelligence-common-sense-engine, и из видео D. Lenat, “Computers Versus Common Sense”, Google Talks Archive, accessed Dec. 18, 2018, www.youtube.com/watch?v=gAtn -4fhuWA.
336
Ленат отмечает, что компания все лучше справляется с автоматизацией получения новых констант (вероятно, благодаря добыче данных из интернета). См. D. Lenat, “50 Shades of Symbolic Representation and Reasoning”, CMU Distinguished Lecture Series, accessed Dec. 18, 2018, www.youtube.com/watch?v=4mv0nCS2mik.
337
Ibid.
338
Подробное описание проекта Cyc для неспециалистов приводится в главе 4 книги H. R. Ekbia, Artificial Dreams: The Quest for Non-biological Intelligence (Cambridge, U. K.: Cambridge University Press, 2008).
339
Сайт компании Lucid: lucid.ai.
340
P. Domingos, The Master Algorithm (New York: Basic Books, 2015), 35.
341
Из “The Myth of AI: A Conversation with Jaron Lanier”, Edge, Nov. 14, 2014, www.edge.org/conversation/jaron_lanier-the-myth-of-ai.
342
См., например, N. Watters et al., “Visual Interaction Networks”, Advances in Neural Information Processing Systems 30 (2017): 4539–4547; T. D. Ullman et al., “Mind Games: Game Engines as an Architecture for Intuitive Physics”, Trends in Cognitive Sciences 21, no. 9 (2017): 649–665; K. Kansky et al., “Schema Networks: Zero-Shot Transfer with a Generative Causal Model of Intuitive Physics”, in Proceedings of the International Conference on Machine Learning (2017), 1809–1818.
343
J. Pearl, “Theoretical Impediments to Machine Learning with Seven Sparks from the Causal Revolution”, in Proceedings of the Eleventh ACM International Conference on Web Search and Data Mining (2018), 3. Подробнее о каузальных рассуждениях в ИИ см. в работе J. Pearl and D. Mackenzie, The Book of Why: The New Science of Cause and Effect (New York: Basic Books, 2018).
344
Информативная дискуссия о недостатках глубокого обучения приводится в работе G. Marcus, “Deep Learning: A Critical Appraisal”, arXiv:1801.00631 (2018).
345
DARPA Fiscal Year 2019 Budget Estimates, Feb. 2018, accessed Dec. 18, 2018, www.darpa.mil/attachments/DARPAFY19PresidentsBudgetRequest.pdf.
346
Бонгард М. М. Проблема узнавания. – М.: Наука, 1967.
347
Все иллюстрации к задачам Бонгарда, приводимые в книге, взяты из коллекции, собранной на сайте Гарри Фундалиса, www.foundalis.com/res/bps/bpidx.htm, в которую, помимо ста задач Бонгарда, входит множество задач, составленных другими людьми.
348
R. M. French, The Subtlety of Sameness (Cambridge, Mass.: MIT Press, 1995).
349
Одну особенно интересную программу для решения задач Бонгарда создал Гарри Фундалис, аспирант из исследовательской группы Дугласа Хофштадтера в Индианском университете. Фундалис подчеркнул, что создает не “решатель задач Бонгарда”, а “когнитивную архитектуру, вдохновленную задачами Бонгарда”. По заветам Бонгарда программа ориентировалась на человеческое восприятие на всех уровнях, от низкоуровневого зрения до построения абстракций и аналогий, но успешно решала лишь небольшое число задач Бонгарда. См. H. E. Foundalis, “Phaeaco: A Cognitive Architecture Inspired by Bongard’s Problems” (PhD diss., Indiana University, 2006), www.foundalis.com/res/Foundalis_dissertation.pdf. Фундалис ведет интересный сайт, на котором рассказывает о своей работе с задачами Бонгарда: www.foundalis.com/res/diss_research.html.
350
S. Stabinger, A. RodrÍguez-Sánchez and J. Piater, “25 Years of CNNs: Can We Compare to Human Abstraction Capabilities?”, in Proceedings of the International Conference on Artificial Neural Networks (2016), 380–387. Схожее исследование с подобными результатами описано в работе J. Kim, M. Ricci, and T. Serre, “Not-So-CLEVR: Visual Relations Strain Feedforward Neural Networks”, Interface Focus 8, no. 4 (2018): 2018.0011.
351
Говоря “большинство людей”, я ссылаюсь на результаты опросов, которые проводила при подготовке своей диссертации. См. M. Mitchell, Analogy-Making as Perception (Cambridge, Mass.: MIT Press, 1993).
352
Хофштадтер предложил термин “концептуальный переход” при обсуждении задач Бонгарда в главе 19 книги D. R. Hofstadter, Gödel, Escher, Bach: an Eternal Golden Braid (New York: Basic Books, 1979). (Русское издание: Хофштадтер Д. Гёдель. Эшер. Бах: эта бесконечная гирлянда / Пер. с англ. М. Эскиной. – Самара: Бахрах-М, 2001.)
353
Ibid., 349–351.
354
Подробное описание Copycat дается в главе 5 работы D. R. Hofstadter and the Fluid Analogies Research Group, Fluid Concepts and Creative Analogies: Computer Models of the Fundamental Mechanisms of Thought (New York: Basic Books, 1995). Еще более подробное описание дается в книге, основанной на моей диссертации: Mitchell, Analogy-Making as Perception.
355
J. Marshall, “A Self-Watching Model of Analogy-Making and Perception”, Journal of Experimental and Theoretical Artificial Intelligence 18, no. 3 (2006): 267–307.
356
Несколько таких программ описано в работе Hofstadter and the Fluid Analogies Research Group, Fluid Concepts and Creative Analogies.
357
A. Karpathy, “The State of Computer Vision and AI: We Are Really, Really Far Away”, Andrej Karpathy blog, Oct. 22, 2012, karpathy.github.io/2012/10/22/state-of-computer-vision.
358
См. Stanford Encyclopedia of Philosophy, s.v. “Dualism”, plato.stanford.edu/entries/dualism/.
359
Аргументированная философская дискуссия о гипотезе о воплощенном познании в когнитивистике приводится в работе A. Clark, Being There: Putting Brain, Body, and World Together Again (Cambridge, Mass.: MIT Press, 1996).
360
“Automated Vehicles for Safety”, National Highway Traffic Safety Administration website, www.nhtsa.gov/technology-innovation/automated-vehicles-safety#issue-road-self-driving.
361
“Vehicle Cybersecurity: DOT and Industry Have Efforts Under Way, but DOT Needs to Define Its Role in Responding to a Real-World Attack”, General Accounting Office, March 2016, accessed Dec. 18, 2018, www.gao.gov/assets/680/676064.pdf.
362
Цит. по: J. Crosbie, “Ford’s Self-Driving Cars Will Live Inside Urban «Geofences»”, Inverse, March 13, 2017, www.inverse.comford-self-driving-cars-geofences-ride-sharing/article/28876-.
363
Цит. по: J. Kahn, “To Get Ready for Robot Driving, Some Want to Reprogram Pedestrians”, Bloomberg, Aug. 16, 2018, www.bloomberg.com/news/articles/2018-08-16/to-get-ready-for-robot-driving-some-want-to-reprogram-pedestrians.
364
“Artificial Intelligence, Automation, and the Economy”, Executive Office of the President, Dec. 2016, www.whitehouse.gov/sites/whitehouse.gov/files/images/EMBARGOED%20AI %20Economy%20Report.pdf.
365
Это отсылает нас к тому, что Алан Тьюринг назвал “возражением леди Лавлейс” по имени леди Ады Лавлейс, британского математика и писательницы, которая в XIX веке вместе с Чарльзом Бэббиджем разрабатывала аналитическую машину – предложенный (но не воплощенный) проект программируемого компьютера. Тьюринг цитирует леди Лавлейс: “Аналитическая машина не претендует на то, чтобы создавать что-то новое. Она умеет выполнять лишь то, что мы можем ей повелеть”. A. M. Turing, “Computing Machinery and Intelligence”, Mind 59, no. 236 (1950): 433–460.
366
Karl Sims website, accessed Dec. 18, 2018, www.karlsims.com.
367
D. Cope, Virtual Music: Computer Synthesis of Musical Style (Cambridge, Mass.: MIT Press, 2004).
368
Цит. по: G. Johnson, “Undiscovered Bach? No, a Computer Wrote It”, New York Times, Nov. 11, 1997.
369
M. A. Boden, “Computer Models of Creativity”, AI Magazine 30, no. 3 (2009): 23–34.
370
J. Gottschall, “The Rise of Storytelling Machines”, in What to Think About Machines That Think, ed. J. Brockman (New York: Harper Perennial, 2015), 179–180.
371
Из видеолекции “Creating Human-Level AI: How and When?”, Future of Life Institute, Feb. 9, 2017, www.youtube.com/watch?v =V0aXMTpZTfc.
372
A. Karpathy, “The State of Computer Vision and AI: We Are Really, Really Far Away”, Andrej Karpathy blog, Oct. 22, 2012, karpathy.github.io/2012/10/22/state-of-computer-vision.
373
C. L. Evans, Broad Band: The Untold Story of the Women Who Made the Internet (New York: Portfolio/Penguin, 2018), 24.
374
M. Campbell-Kelly et al., Computer: A History of the Information Machine, 3rd ed. (New York: Routledge, 2018), 80.
375
Цит. по: K. Anderson, “Enthusiasts and Skeptics Debate Artificial Intelligence”, Vanity Fair, Nov. 26, 2014.
376
См. O. Etzioni, “No, the Experts Don’t Think Superintelligent AI Is a Threat to Humanity”, Technology Review, Sept. 20, 2016, www.technologyreview.com/s/602410/no-the-experts-dont-think-superintelligent-ai-is-a-threat-to-humanity; и V. C. Müller and N. Bostrom, “Future Progress in Artificial Intelligence: A Survey of Expert Opinion”, in Fundamental Issues of Artificial Intelligence (Basel, Switzerland: Springer, 2016), 555–572.
377
N. Bostrom, “How Long Before Superintelligence?”, International Journal of Future Studies 2 (1998).
378
Hofstadter, Gödel, Escher, Bach, 677–678.
379
Из “The Myth of AI: A Conversation with Jaron Lanier”, Edge, Nov. 14, 2014, www.edge.org/conversation/jaron_lanier-the-myth-of-ai.
380
P. Domingos, The Master Algorithm (New York: Basic Books, 2015), 285–286.
381
Из “Panel: Progress in AI: Myths, Realities, and Aspirations”, Microsoft Research video, accessed Dec. 18, 2018, www.youtube.com/watch?v=1wPFEj1ZHRQ&feature=youtu.be.
382
R. Brooks, “The Origins of «Artificial Intelligence»”, Rodney Brooks’s blog, April 27, 2018, rodneybrooks.com/forai-the-origins-of-artificial-intelligence.